Introduction
Cost overruns caused by unforeseen geological challenges are common place for large infrastructure projects. Thorough ground investigations can reduce this risk, but geotechnical drillings and laboratory test are expensive and time consuming. Airborne electromagnetics (AEM) is a low-cost geophysical method being increasingly used for geotechnical ground investigations. However, extracting engineering parameters from these complex data is challenging. We present a novel approach of extracting depth to bedrock from AEM data using artificial neural networks (ANN) and sparse drillings.
Using synthetic models, we test its theoretical performance and analyse sources of error. We find that geological complexity is the main limitation on performance. We also test the algorithm on real field data from a complex geological setting. Results show that ANNs produce bedrock models that rival the accuracy of manual interpretations by experts and that are markedly more accurate than existing automated resistivity model interpretation methods. Using ANN based bedrock interpretation, one needs 2 to 3.5 times fewer geotechnical drillings (i.e., a reduction of 50–70%) in the early phases of a project compared to ground investigations using only borehole data. Further improvements may be possible with strategic planning of drilling campaigns and careful data pre-processing.
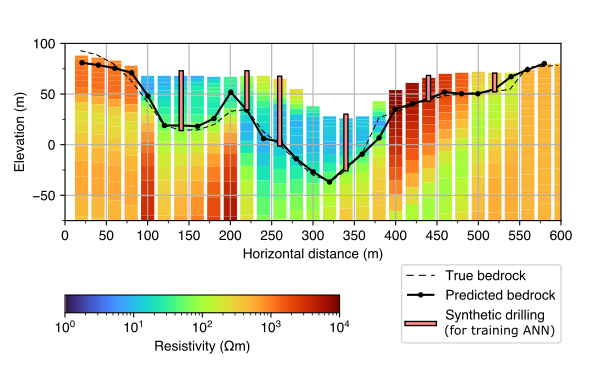
Summary and future directions
In closing, ground models derived from integration of geophysical and geotechnical data via artificial neuronal networks are an enabler for introducing airborne electromagnetic data into engineering project workflows. Our results show that these ground models have clear value for early stages of a development project where limited number of drillings are available. The suggested integration approach reduces uncertainty and can guide engineers in designing the more cost-effective site investigation programs. The method we present aligns well with current industry trends.
The use of 3D models and integrated data management systems like Building Information Modelling (BIM) are widespread in the construction sector (Mehrbod et al., 2019; Tezel et al.,2019). However, the usage of such data management systems in geological and geotechnical engineering is still limited but the obvious benefits have made it an active area of discussion (Svensson, 2019). Being able to acquire a high-resolution bedrock model with good areal coverage early in a project phase with airborne geoscanning will make such 3D workflows more attractive. Moreover, unlike the manual interpretation of the past, having an automated method to quickly update a bedrock model using new borehole information as it becomes available, will maintain the utility of the AEM data throughout a project's lifetime.
Acknowledgments
Parts of the presented work were developed under Research Council of Norway (RCN) FORNY grant “Airborne Geo-Intelligence” (project number 282147). We thank the Norwegian public rail- and road authorities (Bane NOR and Statens Vegvesen) for permission to publish the field data results. The presented findings are the cumulative results of a joint effort over many years to establish helicopter geoscanning within NGI; a big thank you goes to all the colleagues that have been involved.
Reference
The full paper can be requested through the download link above or found directly at www.sciencedirect.com.
Lysdahl, A. K., Christensen, C. W., Pfaffhuber, A. A., Vöge, M., Andresen, L., Skurdal, G. H., & Panzner, M. (2022). Integrated bedrock model combining airborne geophysics and sparse drillings based on an artificial neural network. Engineering Geology, 297, 106484.